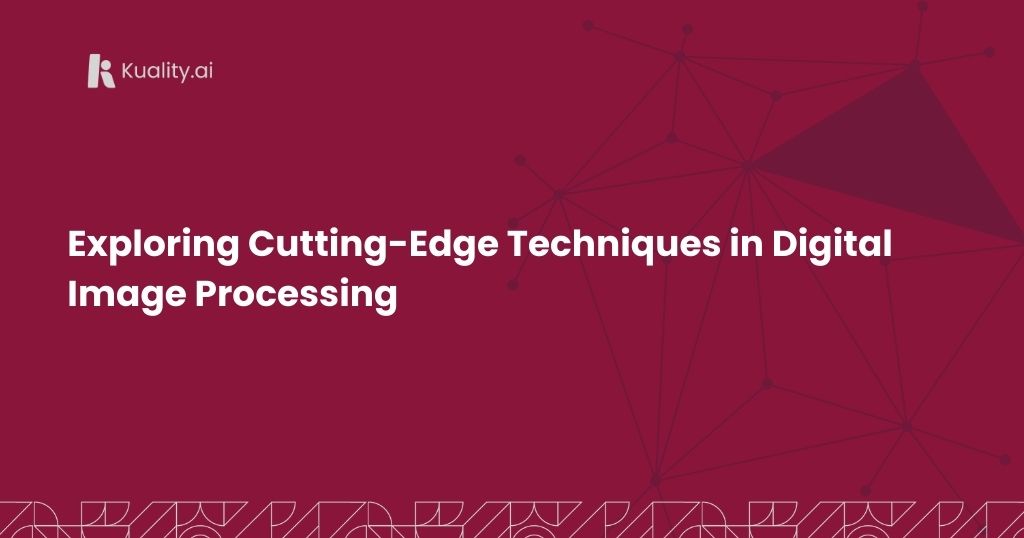
The world as we see it using our visual sense is a wonder to behold, an incredible feat of evolution honed over 500 million years to allow us to appreciate the beauty around us, from a newborn’s smile to the stunning visuals of modern virtual reality.
However, recent technological advancements have allowed us to extend our visual capabilities to machines and computers, enabling them to see and capture the world in new ways.
But while we might take for granted our ability to effortlessly process visual information, machines require a complex set of mathematical algorithms to transform an image into something they can understand. Each image is simply an array of square blocks—pixels—each assigned a numerical value representing its intensity. For grayscale images, each pixel is represented by a single value ranging from 0 to 255, but for color images, there are three channels—red, green, and blue—each containing a value that combines to create the full range of colors.
Understanding Image Processing
Image processing is the technique of applying relevant mathematical operations or algorithms to a digitized image to generate an enhanced image or extract some useful features like edge shape and color. There are various image processing operations that are widely used, including image enhancement, color image processing, image restoration, image segmentation, morphological operations, and object detection.
For example, a simple subtraction operation can be applied to enhance the quality of an overexposed image by reducing its brightness. Similarly, color image processing and image segmentation have very popular applications in the film and television industries. You might have seen a movie or a show being shot with a green screen in the background, which is then replaced with a different video or an image. This is based on simple logic: if a pixel value is equal to the green color intensity, then assign that pixel a value of 0.
Image processing may involve a single-pixel operation or a group-pixel operation. For instance, the effect of bouquet mode, in which the foreground appears sharp and the background is blurred, can be recreated using edge detection and image blurring, which are implemented with the help of the most important phenomenon of image processing called convolution.
Examples of Image Processing Operations
The demand for image processing is increasing across various industries, including medical imaging, automotive imaging, and satellite imaging. With the advancement of technology, diagnostic scans like MRI, ultrasound, and x-rays can be analyzed using image processing and machine learning techniques to detect life-threatening diseases like Alzheimer’s, brain tumors, and cancer at an early stage, which can help save many lives. Many research organizations across the world are doing groundbreaking research in this domain and are also hiring people who are familiar with image processing and machine learning.
Computer vision involves image processing and machine learning to help cars see and comprehend the world around them, which is a vital technology for developing more safe and smart self-driving cars. Many big players in the auto industry are developing technologies to develop such cars, which requires people with an image processing skill set.
Satellite imaging is another area that benefits from image processing, as it helps scientists make critical decisions for the betterment of a planet. For instance, detecting the relative change or analyzing any satellite image involves image processing algorithms, which can help scientists make important discoveries.

In conclusion, the age of information technology has made visual data readily available, but it often requires a lot of processing for tasks like transferring over the internet or extracting insights through predictive modeling. However, with the rise of deep learning technology, convolutional neural network (CNN) models were developed to process images. Since then, many advanced models have emerged that cater to specific tasks in the image processing niche.
From image compression and enhancement to image synthesis, we’ve explored some of the most critical techniques in image processing and the popular deep learning-based methods that address these challenges. But the research doesn’t stop there. Current efforts are focused on advancing the field through innovative concepts such as semi-supervised and self-supervised learning. By reducing the need for ground truth labels for complex tasks like object detection and semantic segmentation, these methods can make models more suitable for a wide range of practical applications.
Overall, the future of image processing is bright, and the ongoing developments in deep learning technology hold great potential for further advancements in the field. With new techniques emerging regularly, we can expect to see even more exciting developments in the coming years.